
Decode the Top 8 Use Cases of Pytourch in the Finance Sector
In the finance sector, having compelling insights is crucial. Here, PyTorch emerges as a transformative force in reshaping financial sectors to navigate various challenges and make essential decisions. PyTorch is an open-source machine learning library known for its innovation, offering exceptional flexibility and a dynamic graph that helps the financial sector's demands for data. In this article, we will discuss the top use cases of PyTorch that excel in the finance industry.
Top 8 Use Cases of Pytourch in the Finance Sector
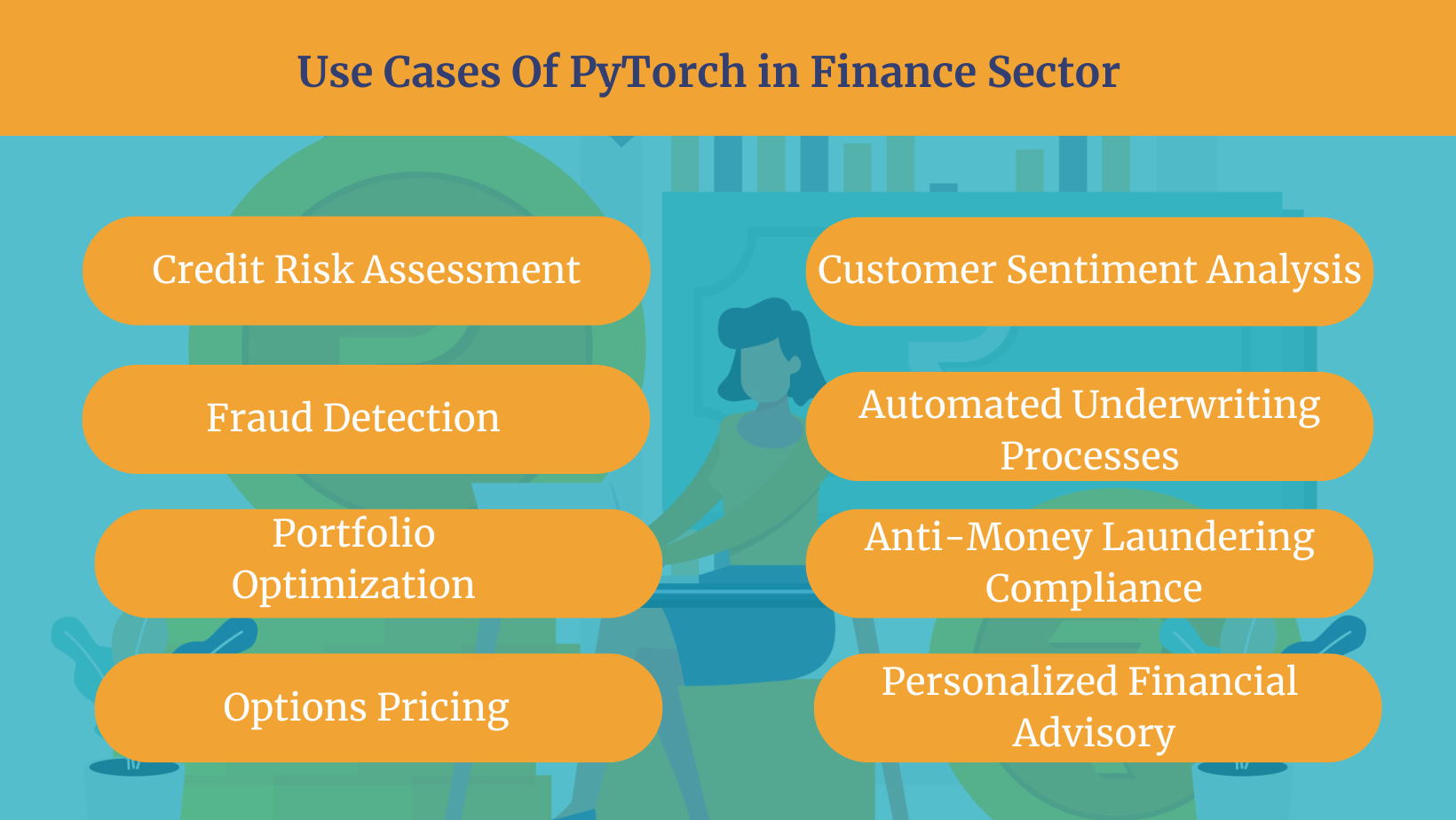
1. Credit Risk Assessment
PyTorch can be used to craft credit scoring models for individuals or businesses for creditworthiness. Using deep learning capabilities can be beneficial for delving into various factors, such as previous financial data and non-traditional data sources. This will help in understanding the complexities of real-life financial scenarios.
2. Fraud Detection
Handling fraud activities is a challenging task for most financial sectors. Hire PyTorch developers who will help in detecting fraud activities. The developer will improve all traditional methods and patterns intricately linked to fraudulent activities. PyTorch is efficient in fraud prevention systems; it acts as a safeguard against potential threats.
3. Portfolio Optimization
Pytourch helps the management to manage their portfolio. It aims to provide a compelling portfolio that not only seeks to maximize returns but also strives to minimize risks. It also analyzes the optimal asset allocations, aligning the investment strategy with both financial goals and risk tolerance.
4. Options Pricing
PyTorch is a powerful tool for creating advanced deep-learning models that can improve the accuracy of options pricing models. It analyzes the previous data and market trends and refines the pricing of products. It also improves pricing accuracy and manages the risks associated with options trading. PyTorch enables business persons to make financial decisions and provide effective insights.
5. Customer Sentiment Analysis
With the help of natural language processing(NLP), PyTorch opens the door to analyzing sentiments within financial news, social media, and other data. This will help financial professionals to understand the market effectively, gain fine insight into market sentiment, and go beyond additional analyses.
6. Automated Underwriting Processes
PyTorch is transforming the insurance industry by automating underwriting processes. It can even analyze a wide range of data, including customer profiles, medical histories, and risk factors, with the help of deep learning models. With this, it becomes easy to understand underwriting complexities. The insurance sector can boost accuracy and efficiency, streamlining operations.
7. Anti-Money Laundering (AML) Compliance
PyTorch, with its advanced capabilities, has become an essential tool in fighting against money laundering and is at the forefront of Anti-Money Laundering (AML) efforts. PyTorch enables the detection of fine and complex activities associated with money laundering. It even has the ability to handle vast datasets and reveal them to financial institutions.
8. Personalized Financial Advisory
PyTorch can help the financial sectors by providing financial guidance. It also offers tailored suggestions for investment strategies, savings plans, and overall financial planning. It supports individual preferences, risk tolerance, and financial aspirations, and generic recommendations are effective. It also prepares a personalized roadmap and strategies with personal goals for an effective financial planning experience.
Summary
PyTorch is a powerful and versatile tool that has proven to be highly effective in the finance sector. It has enabled innovation and efficiency across various critical applications. It shows its effectiveness in portfolios, predicts economic indicators, enhances automation, and fortifies anti-money laundering. However, by leveraging it, financial sectors can make data-driven decisions.